Clustering and Manifolds - Outlier Detection
Algorithm Features
- No parameters to determine: All parameters -- Kernel-sigma, alpha and the number of clusters can be automatically determined.
- Competitive results with existing methods such as K-Means and Spectral Clustering.
- Assigning points to Clusters with respect to the underlying intrinsic structure of the data.
- Outlier Detection: The method automatically determines outliers in the data set. This can lead to additional insights. In the paper we compare our method with Spectral Clustering.
- Out-of-sample:New data-points can be assigned to an existing cluster model without rebuilding the model.
- Successfully applied to real world data: handwritten digits (USPS), Yale Face Database B, Robot Data. The algorithm works well for image data that has an underlying intrinsic structure.
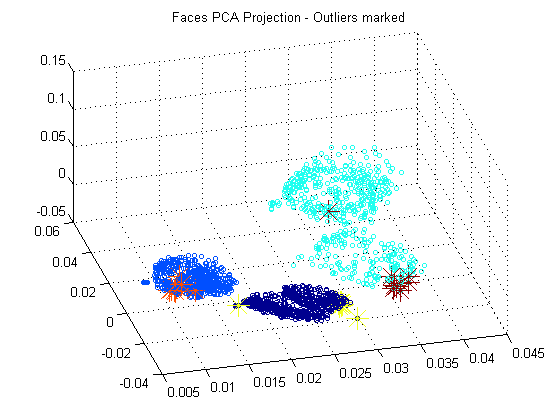
Outlier Detection with Yale Face Database B: PCA-projection of the photographs (1200 dimensional). The outliers the algorithm identified have been marked and are at the most outside points of the blobs.
To do...
- Feature selection - If too much noisy features are present, the underlying intrinsic structure might not be found.
- Scalable - The current method is slow though it's abilities for clustering should be applicable to practical Data Mining tasks.
Papers
- Clustering Through Ranking On Manifolds , ICML 2005
- Clustering with Local and Global Consistency - Available as Technical Report CU-CS-973-04
Code
The Matlab code from our paper is available here.
Links
Links to other interesting clustering stuff on the web.